Computational Approaches To Navigating Network Biology
By Caleigh Findley
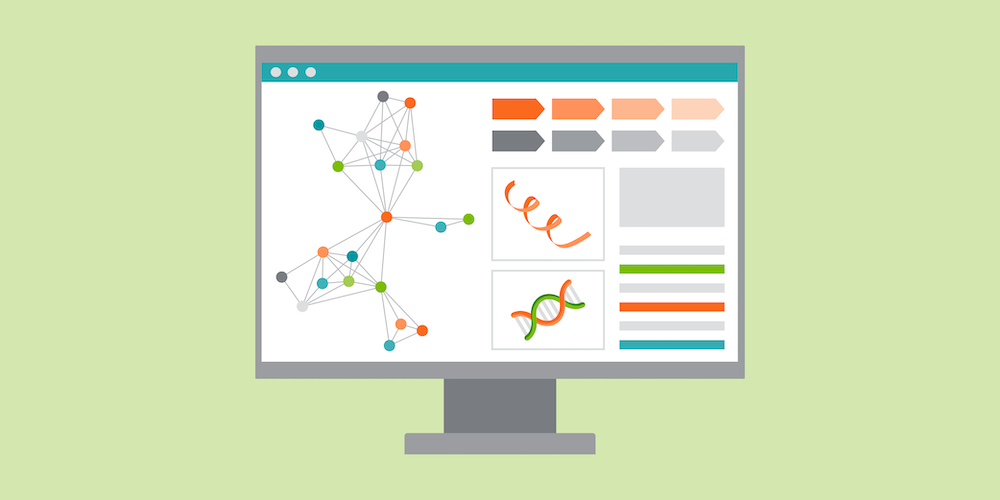
Network biology has become increasingly essential in modern science. With the vast amount of data generated from genomic, proteomic, and metabolomic studies, traditional analyses are no longer sufficient. Powerful tools have emerged to better organize and understand these datasets—enabling researchers to model, analyze, and visualize biological networks with unprecedented precision.
Read on to learn more about some of the computational approaches used in network biology.
The Problem: Finding Tools to Fit the Job
Network biology looks at the greater picture. It examines how different components (genes, proteins, etc.) and molecular interactions create a cellular signaling pathway that underlies a greater biological function. These communication systems can become incredibly complex and difficult to tease apart without specialized software.
Using computational methods makes the sheer volume and intricacy of the biological data used in network analyses more manageable. These approaches enable researchers to integrate diverse data, predict interactions, and analyze biological networks.
The Solution: Computational Techniques in Network Biology
Network Reconstruction and Visualization
Reconstructing biological networks requires compiling interaction data to build models that reflect biological processes. Tools like Cytoscape and Gephi provide powerful visualization capabilities, allowing researchers to see the complex interplay of cellular components.
Pathway Enrichment Analysis
This technique identifies which biological pathways are significantly represented in a given dataset. Tools like DAVID and Reactome use statistical methods to pinpoint pathways with potential relevance to specific conditions or diseases.
Gene Co-expression Networks
Gene co-expression networks map genes that show similar expression patterns across different conditions or tissues. Techniques such as Weighted Gene Co-expression Network Analysis (WGCNA) help identify modules of co-expressed genes and their relationships to phenotypic traits.
Protein-Protein Interaction (PPI) Networks
PPI networks map the physical interactions between proteins. Databases like STRING and IntAct curate known interactions, while predictive tools use computational models to suggest new interactions, aiding in discovering novel protein functions and pathways.
Metabolic Networks
This approach maps the network of biochemical reactions within a cell. Tools like KEGG and MetaCyc provide databases and computational tools to explore metabolic pathways, offering insights into cellular metabolism and its regulation.
Applications of Network Biology
Investigating Disease Mechanisms
Researchers can identify potential therapeutic targets by mapping the networks involved in disease processes. For example, cancer research has benefited from network biology by revealing key genes and pathways involved in tumor development and progression.
Drug Discovery and Repurposing
Network-based approaches can identify new uses for existing drugs by uncovering their effects on various pathways. This repurposing accelerates the drug discovery process and reduces development costs.
Personalized Medicine
Network biology enables the integration of multi-omics data to understand individual variations in disease pathways. This knowledge facilitates the development of personalized treatment plans tailored to each patient’s genetic makeup.
Synthetic Biology
Designing synthetic biological systems requires a deep understanding of cellular pathways. Network biology provides the frameworks needed to predict the behavior of engineered biological systems and ensure their function as intended.
Conclusion
Computational approaches in network biology offer several benefits, including efficiency, comprehensive analysis, and predictive power. Automated data integration and analysis saves time and resources compared to traditional experimental methods. These methods can also analyze large datasets, revealing insights that might be missed with smaller, more focused studies. Further, computational models can predict interactions and behaviors, guiding experimental research and reducing trial-and-error.
Embracing these computational techniques allows researchers to navigate the complex web of cellular interactions with greater precision and depth, paving the way for groundbreaking discoveries and innovations in the life sciences.